Dietmar Paier, UAS BFI Vienna
These guidelines were developed in work package 3 of the AI-HED project. The author expresses his gratitude and appreciation to all experts and to all members of the AI-HED project who have greatly contributed to the development of this document. Any shortcomings or errors remain the sole responsibility of the author.
Experts:
Expert | Affiliation |
---|---|
Jurica Babić | Associate Professor, University of Zagreb, Faculty of Electrical Engineering and Computing |
Philippe Borremans | Crisis & Emergency Risk Communication Consultant; Vice President, International Association of Risk & Crisis Communication (IARCC) |
Adriana Cardoso | Teacher, Polytechnic University of Lisbon, Lisbon School of Education |
Martina Tomičić Furjan | Associate Professor; Vice Dean, University of Zagreb, Faculty of Organization and Informatics |
Anna Füssl | Head of Service Unit of Process Management in Teaching Development, Technical University Vienna |
Meagan Klaij | Education Specialist, Microsoft Netherlands |
Barbara Maly-Bowie | Teaching and Learning Expert, University of Applied Sciences BFI Vienna |
Iris van der Meer | Educational Advisor of Blended Learning, Amsterdam University of Applied Sciences, Faculty of Business and Economics |
Vítor Santos | Researcher, Polytechnic University of Lisbon |
Markus Schatten | Full professor, University of Zagreb, Faculty of Organization and Informatics |
Frederik Situmeang | Associate Professor AI & Data-Driven Business, Amsterdam University of Applied Sciences, Faculty of Business and Economics |
Jeroen StrijBosch | Education Specialist, Microsoft Netherlands |
Barbara Maly-Bowie proofread the text and provided valuable suggestions for improvement.
Erasmus+ Cooperation partnership: Artificial Intelligence in Higher Education Teaching and Learning (AI-HED)
Project number: 2024-1-NL01-KA220-HED-000248874
Disclaimer: Funded by the European Union. Views and opinions expressed are however those of the author(s) only and do not necessarily reflect those of the European Union or the National Agency Stichting Nuffic. Neither the European Union nor the granting authority can be held responsible for them.
1. Objectives
These guidelines particularly address teachers who have a strong interest in exploring the strengths and opportunities of AI in academic education, while also considering potential risks and avoiding threats.
Although the focus is on generative AI, these guidelines also refer to topics relevant to other types of AI: Rethinking teaching concepts, the need for advancing teaching skills, ensuring accessible, equitable, and inclusive education, legal compliance and ethical responsibility in using AI for teaching, learning, and research are topics relevant in every academic area.
These guidelines have three primary aims:
-
to increase teachers' knowledge of the implications of using AI in academic education,
-
to highlight didactic design options, teaching scenarios, and requirements for realizing the positive potential of AI in academic teaching while avoiding unintended effects in courses,
-
to support the legally compliant and ethically responsible use of AI in university teaching.
Delimitation:
These guidelines do not provide information and guidance on practical teaching methods, e.g. which AI tool is best suited for a particular teaching purpose, advising students on formulating meaningful prompts, or evaluating AI output.
For detailed information on the practicalities of teaching with AI, the AI-HED project is developing a comprehensive AI STARTER KIT which provides valuable information on AI terminology, teaching concepts, a heatmap of AI tools suited for promoting specific skills, a well-documented list of recommendable AI tools, and a collection of good practices in teaching with AI.
2. The impact of AI on academic education
2.1 AI as game-changer
The public release of ChatGPT-3.5 at the end of 2022 sparked enormous interest in AI. Within a very short time, the potential applications of AI in academic education, its opportunities, and its risks became central topics in everyday discussions about academic teaching, learning, and research.
Of course, universities have been integrating digital tools into teaching and learning for decades. Just consider the rise and continuous adoption of e-learning since the late 1990s.
However, AI is fundamentally different. The rapid proliferation of AI applications since 2023 has highlighted an unprecedented intertwining of education and technology, profoundly affecting teaching, research, and academic integrity. In response, universities started to invest significant resources to adapt to these developments and to adopt AI in teaching, learning, and research, e.g. by publishing institutional policies on how to embrace AI (cf. MacDonald 2025, Lee et al. 2024).
Many questions and uncertainties about the benefits and risks of AI have been prompted: How should teaching and assessment practices evolve? To what extent are traditional educational models being disrupted - potentially for the better? How can teachers use AI to enhance teaching and learning? How can we ensure the responsible and ethical use of AI?
The possibilities for integrating AI into teaching are extensive, as detailed for generative AI in Chapter ‘5. Designing courses’ of these guidelines.
Regardless of this, one thing is beyond doubt: ignoring AI in teaching and learning in higher education is not an option. Teachers must embrace its potential to enrich, integrate, or fully transform learning environments to ensure that AI’s game-changing opportunities align with pedagogical values and meet the evolving needs of students, and society.
2.2 Rethinking courses
Using AI for teaching & learning requires addressing three fundamental pedagogical questions:
-
How do learning outcomes need to be reformulated?
-
How must teaching materials, learning exercises, and assignments be adapted, developed further, or enhanced?
-
Which methods and criteria are to be applied to assess the work and examinations that students complete with AI?
These questions are directly connected with fundamental issues of academic education which inevitably arise when AI is used. The most important issues include
-
the use of AI by the principles of academic integrity,
-
the legally compliant use of AI,
-
and the ethically responsible use of AI.
While the core principles of academic integrity, legal compliance, and ethical integrity are the same across disciplines, the practical implementation of teaching methods varies considerably between academic faculties and disciplines.
2.3 Advancing teaching skills
Teachers need AI literacy and skills to support students in developing AI literacy and skills. Within the wide scope of AI, certain skills are essential, such as prompting, using AI in a legally compliant and responsible manner, generating teaching materials or assessments with AI, and critically evaluating AI outputs (cf. Lee 2023). Thus, teachers are encouraged to maintain and expand their roles and skills as architects of meaningful learning environments by embracing professional development in the age of AI (see Chiu 2024). This will enable them to design pedagogically, legally, and ethically sound scenarios in which AI serves as a teaching and learning tool.
Check your areas of AI expertise
For teachers, adopting AI means acquiring skills at four levels:
-
The ability to use specific AI tools, general and subject-specific, as standard elements of a professional teaching skills portfolio (technical AI skills).
-
The ability to guide and advise students in the professional, legally compliant and ethically responsible use of AI tools and in critically evaluating outputs (AI teaching skills).
-
The ability to use, customize, or develop AI tools like chatbots for teaching and learning (AI development skills).
-
The ability to develop and apply methods for assessing students’ progress, works, and examinations accomplished with AI (AI-related assessment skills).
Building one’s own digital and AI literacy, such as identifying AI's potential and limitations, and devising strategies for its integration into teaching practices, is imperative to leverage it. To this end, the AI-HED project has developed workshops and interactive AI-HED Training Materials for Teachers.
Enhance your prompting skills
Prompting is the primary method of communicating with generative AI tools. A prompt is an instruction or input provided to an AI system to elicit a specific response. Producing high-quality outputs often requires a structured, step-by-step approach. The practice of giving systematic instructions to generative AI is known as ‘prompt engineering’, which involves specialized techniques and methods to develop and optimize prompts effectively.
Prompting and prompt engineering are essential components of teaching skills in two ways:
-
First, they are necessary for creating course materials, assignments, exams, and other teaching-related content using AI.
-
Second, teachers must be able to guide students in using prompts effectively. This entails understanding the types, techniques, and quality criteria of prompts and prompting.
AI companies usually provide valuable prompting and prompt engineering guides (e.g. Anthropic 2023, OpenAI 2023) with explanations of key terms, techniques, practical examples, and more.
For teachers seeking an easy introduction to prompting, a growing number of universities and educational institutions offer useful resources and examples, such as:
-
Introduction to basic concepts of AI, elementary guidelines, and quickstart resources (metalab at Harvard 2025)
-
Prompt libraries featuring examples from academic teaching across a wide range of subjects (e.g., Maastricht University 2024, Wharton University n.y.)
-
Prompt labs for generative AI in university teaching (e.g. AI Campus 2024, available in German)
-
Prompts and example outputs for various tasks, including developing research questions, writing code, and creating assessments (e.g. University of Edinburgh 2024)
Stay up to date with the latest developments in AI – in academic teaching and the workplace
Some applications of AI are well established, while many others are still emerging. As AI is adopted in more and more areas of professional, public, and private life, teachers must stay informed about new tools and concepts. Teachers should also explore ways to integrate AI with other learning technologies and leverage innovative applications in their courses.
Staying up to date requires understanding how AI is being utilized in the occupational fields where students are expected to work. To this end, universities have adopted particular strategies, e.g. carrying out analyses of AI-related qualification requirements and providing this information to teachers and academic experts, for purposes of program and course development.
Irrespective of this, there are various easy ways to keep up to date for teachers:
-
seek advice from experts from the relevant professional field
-
analyse which specific AI skills are cited in typical job announcements
-
take a look at the AI-HED AI Tools Heatmap.
Ultimately, there is one simple recommendation for educators considering AI adoption: Dive in!
2.4 Types of AI
The term ‘generative AI’ is arguably the most well-known type of AI, yet it is often mistakenly equated with artificial intelligence as a whole. In academic education, a wide range of AI systems and tools are relevant that not primarily aim at producing new content like generative AI but focus on identifying patterns in data to make predictions or decisions. Here is a selection of types that are used in academic education for different purposes.
Generative AI uses frameworks and models to produce new content, such as text, images, sound, or code. To this end, generative AI uses models like large language models (LLM) to learn patterns, structures, and relationships within the data, which are then used to generate new content. Teachers use generative AI to develop teaching and assessment materials such as presentations, rubrics, educational videos, assignments, and tests.
Predictive AI uses algorithms and architectures to forecast future outcomes by analysing historical trends and correlations. Predictive AI rapidly gains importance in various disciplines, e.g. for business analytics, forecasting disease progression in health care, or protein structure prediction in biology. In higher education, prediction of student retention is a typical field of application of predictive AI.
Assistive AI focuses on models and frameworks designed to support human decision-making or tasks without necessarily generating new content. In higher education, assistive AI is applied to various functions, including research paper analysis, text corrections and improvements, grading assistance, administrative task automation, and enhancing accessibility for students with disabilities.
Adaptive AI adjusts algorithms and models dynamically, learning from new data or environmental changes to improve performance. In higher education, adaptive AI can personalize learning paths by analysing student performance and dynamically tailoring content to individual needs.
Simulative AI employs models and computational architectures to create virtual environments or scenarios that mimic real-world processes for training, testing, or experimentation. In higher education and research, it is used for virtual labs, research simulations, engineering design testing, and modeling of climate models or molecular interactions.
3. Pedagogical approaches towards adopting AI in academic education
3.1 AI as a tool
A primary purpose of using AI in academic education is for students to use AI as an aid and as a tool for achieving learning objectives. Teachers need to decide how to use the affordances of AI as a tool, as the didactical value of technology is never inherent but must be thoughtfully developed with the learning outcomes for students in mind.
Therefore, AI is a tool to be utilized; it is not AI that dictates the practice of teaching and learning, but the teacher who determines its relevance and functions within their course.
3.2 Human-centered course design
Teachers and students are at the forefront of increasingly technology-driven learning environments. While AI can enhance and expand pedagogical possibilities, it cannot replicate the nuanced understanding, empathy, and mentorship that educators bring to the learning process. A human-centered approach ensures that teachers remain integral as learning architects and coaches using AI at best to cultivate curiosity, promote higher-order thinking, and support the emotional and social dimensions of learning across diverse educational scenarios.
Following a human-centered approach in academic teaching means designing courses in a way that supports the involvement of learners in all stages of the learning process according to prior knowledge, capabilities, and needs in relation to defined learning outcomes. In all steps of designing and developing a course, the needs of learners remain the key reference of didactic design.
AI offers a wider range of opportunities to tailor courses more effectively to the interests, strengths, and needs of students.
3.3 Accessible, equitable, and inclusive education
However, AI presents both threats and opportunities. To support students in developing professional, creative, and responsible use of AI as a key skill of this decade, every pedagogical approach must be grounded in three principles: accessibility, equity, and inclusion.
Ensure equal access for students
Accessibility ensures that all learners, including those with disabilities and other learning challenges, have equal opportunities to achieve learning outcomes by accessing and engaging with course materials, activities, and assessments equitably, regardless of personal, social, or economic circumstances. This also means that students from lower-income backgrounds must not face disadvantages when using AI in academic education.
Take care of diversity and equity
Equity refers to transforming educational practices and using AI to actively support students from diverse backgrounds, with varying language skills, prior knowledge, and learning habits. The goal is to provide equal opportunities for all students to achieve learning outcomes.
AI tools should facilitate, not hinder, diverse learning needs. Consider how these tools can be adapted to accommodate various backgrounds, abilities, and language proficiencies, fostering inclusive learning experiences that respect different learning habits, backgrounds, perspectives, and approaches while preventing disadvantages stemming from personal, cultural, social, or economic circumstances.
Design inclusive teaching and learning
Inclusion is defined as “an ongoing process aimed at offering quality education for all while respecting diversity and the different needs and abilities, characteristics and learning expectations of the students and communities, eliminating all forms of discrimination” (UNESCO 2009: 126). This definition clarifies that using AI is a necessary step, but not a sufficient one, and that teachers must actively reframe teaching and learning practices to create learning environments where all students can equally develop AI skills.
Therefore, consider students’ varying levels of access to technology, too. Use AI options that promote equal access to AI tools for learning, assignments, and assessments in your course to avoid disadvantaging students who may have limited resources.
4. Principles of course design
4.1 Integrating AI into the Constructive Alignment Approach
Constructive alignment is an effective technique to create pedagogically sound courses. It means systematically aligning the core elements of teaching - learning outcomes, learning activities, and assessment - with one another. When integrating AI into a course, it is crucial to think about the purpose of AI and how students can best utilize it to achieve the intended learning outcomes.
Teachers are recommended to follow three key steps in course design:
1. Define the AI-related elements of learning outcomes in terms of assessable knowledge, skills, and competencies. This is the basis for selecting course content.
2. Establish the criteria and methods for assessing the achievement of AI-related learning outcomes.
3. Select appropriate AI tools and determine how students will use them in exercises, assignments, presentations, examinations, and other activities.
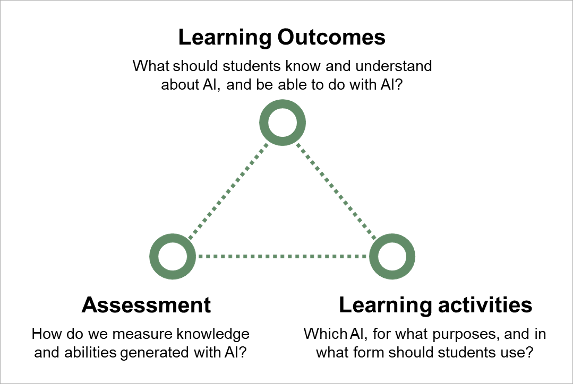
Integrating AI into Constructive Alignment
When applying the constructive alignment approach to course design, it is helpful to distinguish between different kinds of AI-related learning outcomes:
-
AI knowledge and skills may be defined as direct learning outcomes, e.g. when students are expected to describe the components of AI systems, to explain how they are connected, or to develop an AI system.
In this case, AI knowledge and skills are subject-specific, and the learning outcomes focus on professional, methodical, or analytical competencies.
-
Students might be advised to use AI as a tool that helps them achieve an overarching learning outcome, e.g. using a specific AI tool to summarize texts and to extract relevant content to produce an overview of the state of the art in a given subject.
In the second case, a variety of AI tools can be used to help achieve a higher-order learning outcome. In relation to this learning outcome, the use of AI can be considered an important element of general AI literacy, and therefore a transversal competence.
This distinction is important because it influences both the emphasis placed on each learning activity during assessment and the criteria used to evaluate it.
4.2 Cultivating critical thinking
Critical thinking essentially means the ability to interpret, analyse, and evaluate information, facts, and behaviour independently, reflexively, and sceptically. This means being able to make a ‘purposeful, self-regulatory judgment which results in interpretation, analysis, evaluation, and inference, as well as explanation of the evidential, conceptual, methodological, criteriological, or contextual considerations upon which that judgment is based’ (Facione 1990: 2).
AI tools generally generate incomplete and often incorrect, distorted, or misleading outputs, the ability to critically scrutinize AI-generated outputs is gaining particular importance. Therefore, teachers need to advise and train students to critically assess AI outputs, verify facts, and recognize biases, preparing them to discern accurate information. See Chapter ‘5.4 Teaching strategies to promote critical thinking’ for practical ideas to support students’ critical thinking.
4.3 Fostering academic integrity
With generative AI tools capable of generating text, images, sound, and code, academic institutions need to re-emphasize the relevance of the independent work of students, teachers, and researchers. This shift necessitates the development of academic integrity policies focusing on disclosing AI use, distinguishing between human and AI-generated content through proper documentation, quotation, and acknowledgment of outputs generated by AI as basic requirements.
A promising approach towards ensuring the transparent use of AI is to require teachers, students, and researchers to document their use of AI and to explain how, and to what extent, AI-assisted or AI-generated data and output were used in preparing assignments, theses, or research reports.
For handling academic integrity in the course, see Chapter ‘5.7 Demanding academic integrity and good scientific practice’.
5. Designing courses: Didactical scenarios, teaching methods, assessment & grading
5.1 AI for student-centered and personalized learning
Embrace AI as a co-teacher – and as a learning partner for your students
Students can use AI as a study tool, tutor, brainstorming partner, and much more. Teachers must consider how students should interact with AI tools and AI-driven resources. To this end, teachers need to be aware of the many ways in which AI can be used and get familiar with the idea that AI can be made a valuable co-teacher. Here are a few examples of how teachers can adopt AI as co-teacher.
For instance, teachers should seek using generative AI for
-
to create tailor-made learning materials and feedback, e.g. to support personalized learning paths, to support students with minor prior knowledge closing knowledge gaps, or to support or to motivate particularly talented students with extra tasks,
-
to create challenge-based, game-based, and real-world learning environments to motivate students to engage more with the contents,
-
or to provide real-time feedback and recommendations based on personal learning progress.
Embracing generative AI as a learning partner for students includes designing and training chatbots and integrating them into courses as ’learning buddies’, tutors, feedback providers, and more.
At a more advanced level of AI proficiency, analytical and predictive AI tools can be deployed deployed to analyse student engagement patterns, and to predict students-at-risk or student success.
Think about how to use AI for more personalized and adaptive learning
AI can help tailor learning paths to individual student needs, enabling personalizing feedback, learning processes, and learning materials. This allows students to grasp complex topics at their own pace, catering to different learning speeds and styles. While teachers are often accustomed to designing interaction with students for a uniform group of students, AI provides many opportunities to adapt teaching and learning processes to diverse needs and interests.
Define the purpose and role of AI in your course
Generative AI can be used for multiple purposes and in many different ways in courses and, thus, may take very different roles for students. As a teacher, you should be very clear about both.
AI can take very different roles (see Mollick & Mollick 2023):
-
Mentor: Provides frequent, immediate, and adaptive quality feedback to support student learning.
-
Tutor: Uses questioning techniques, collaborative problem-solving, and personalized instruction to guide students.
-
Coach: Helps students understand learning processes, identify gaps in their knowledge, and develop effective study techniques.
-
Teammate: Contributes specific knowledge or skills to a team, offers social support, and presents diverse perspectives.
-
Simulator: Facilitates skill and knowledge transfer by creating contexts of application, AI-based agents, and settings with distributed roles.
-
Tool: Assists in carrying out developmental, analytical, evaluative, design, creative tasks.
5.2 Didactic scenarios for teaching with generative AI
There are numerous didactical scenarios for integrating AI into a course. Below is a – highly selective – list of teaching concepts with some practical indications that may be useful when preparing a course.
Pedagogical Approach | Implementation |
---|---|
Problem-based Learning (PBL) | PBL is a practice-oriented teaching and learning method in which students work autonomously on authentic problems in small groups in a self-directed manner, while teachers supervise them as tutors. AI can assist in the development of problem-solving skills through: - Search for information about problem structure and manifestations. - Discussing and evaluating problem-solving techniques. - Evaluating pros and cons of problem-solving approaches. - Providing expert knowledge for specific tasks and challenges. |
Project-oriented Learning (POL) | POL fosters collaboration to develop and implement solutions to real-world problems. AI may: - Act as a virtual collaboration partner. - Suggest project team formations based on expertise. - Act as a coach to improve collaboration quality. - Examine the progress and quality of project work. |
Constructive Feedback & Coaching | Constructive feedback reinforces positive learning achievements and reformulates errors as areas for improvement. AI can: - Provide personalized feedback. - Assist students in self-assessment. - Reinforce and motivate students. |
Discourse-oriented Learning | Acquisition of knowledge can be achieved through formal or informal exchange of perspectives. AI can: - Use chatbots to represent different perspectives in discussions. - Act as a Socratic dialogue partner. - Let students test their opinions against a virtual sparring partner. |
Linking Theory and Practical Application | Integrating theory with practice enhances understanding. AI can: - Visualize linkages between theoretical concepts and practical challenges. - Explore AI’s applicability in real-world settings. |
Self-directed Learning | AI helps learners by: - Identifying learning needs. - Organizing individual learning paths. - Searching and evaluating learning resources. - Documenting and reflecting on progress. |
Game-based Learning (GBL) | AI can take roles in game-based learning as: - Game co-developer. - Game character. - Teammate. - Consultant for game strategy. - Referee. |
Critical Thinking | AI requires critical evaluation due to potential inaccuracies. AI can: - Encourage students to verify factual accuracy. - Support reflections on AI-generated outputs. - Foster awareness of AI’s influence on perceptions and work processes. |
Table 1: Didactic scenarios of teaching with AI
5.3 Anticipating potential implications of AI for your course
Reflect on the impact of AI on course-related time management
Whichever teaching scenario you choose, using AI in the teaching setting often requires more time than expected: Teachers must allocate time for explaining the purpose and roles of AI, the rules of usage, assessment criteria, and providing instructions on smart prompting, prompt evaluation, as well as fostering critical reflection and evaluation of AI outputs. As we know from previous experience, the time required to clarify AI-related questions with students is often underestimated.
These activities cannot be done casually, and more time instructing students on using AI and evaluating outputs should be scheduled when designing a course. As the number of teaching units usually remains unchanged and working with AI is an add-on, teachers may need to adjust the course timeline to ensure sufficient time for AI-related activities.
Anticipate changes in learning behaviour triggered by AI
AI may affect learner behaviour in many ways. When assessing the potential implications, consider how AI can positively impact student learning and how to mitigate possible drawbacks. To better anticipate changes, it often helps to complete assignments that require AI use yourself or discuss this task with colleagues to identify potential challenges and improvements, too.
Rethink the quality of learning outcomes
If students are encouraged or required to use AI for assignments and examinations, teachers should establish criteria to assess the results when part of them is AI-generated. Two options are particularly relevant: First, define assessment criteria for correct, responsible, and successful use of AI itself; and second, define assessment criteria for independent parts of students’ work, e.g. critical reflection of the quality of outputs and using outputs for other tasks.
5.4 Teaching strategies to promote critical thinking
Always link the use of AI with critical thinking:
AI can do parts of the work we have done ourselves before. Generative AI in particular often produces false or misleading output. Therefore, students need to learn how to evaluate this output critically. This can be done with or without the help of AI.
Students may be assigned
-
to evaluate outputs regarding factual accuracy, consistency, and academic integrity in the classroom without the help of digital media
-
to reason and reflect on the relevance and impact of AI-generated outputs for particular groups in terms of diversity, inclusion, and sustainability
-
to reflect on the risks that arise when AI-generated outputs are adopted without critical assessment
-
to reflect on the ways the use of AI is shaping our results, how we perceive reality, and the way we work and collaborate with others
To develop students’ ability of critical thinking, some teaching methods have proven to be particularly helpful and effective. One common element of these methods is to test the output against other sources of information and knowledge.
Method | Approach | Implementation |
---|---|---|
Observe-Question-Compare (OQC) | Analyzing an AI output by examining its details and comparing its information with an authoritative source | Observe: Identify and examine the features of the AI output, even if they feel self-evident. For instance, ask: “How many different aspects are addressed in the output?” Question: Critically evaluate every aspect of the output by asking questions such as: Is the AI-generated information true and accurate? Is it relevant? Is it fair? Compare: Let students compare AI output with other credible sources of information, e.g., textbooks, journal articles, descriptions of good practices, professional association information, or market research data. |
Review-Evaluate-Reprompt (RER) | Defining the quality standards for a specific task and evaluating AI outputs based on those criteria. | Review Criteria: Define (also in collaboration with your students) what constitutes a quality, desirable, or “good” output. This could include specificity, context, organization, clarity, usability, format, limitations, and other factors. Evaluate: What are the gaps between the defined criteria and the AI-generated output? What has the AI added, deleted, or modified? What advice does it offer for improvement, based on the feedback it provides? What might AI not be able to improve, given its inherent limitations? Refine the Prompt: Use the identified criteria to craft more detailed or specific instructions for the AI. Regenerate the output with a refined prompt, or decide to stop prompting and make the necessary changes manually. |
Ideas-Connections-Extensions (ICE) | Generating ideas, making connections between them, and extending them into new applications. | Ideas: Generate initial ideas on the questions to be answered or problems to be solved using AI. For example, what potential solutions could help reduce plastic waste in the community? Connections: Identify and explore connections between these ideas and the insights gained from AI. For example, how can these solutions be integrated with existing recycling programs or community initiatives? Extensions: Extend these ideas into broader applications using AI for further research. For example, how can these solutions be scaled up or adapted for other environmental issues? |
Table 2: 3 Approaches to Critical Thinking (from Paulson 2024, with minor adaptations)
5.5 Using AI for feedback to students
Imagine a co-teacher who is available to students for feedback 24/7. Teachers may use AI as a co-teacher that provides real-time feedback on assignments, quizzes, and essays, allowing students to identify areas for improvement immediately, without waiting for personalized feedback. This instant feedback loop promotes continuous improvement and engagement.
AI can be used to design assignments, develop examination methods, and refine assessment criteria.
AI could also assist teachers in grading multiple-choice exams, providing rubric-based feedback on essays, and assessing assignments, and other tasks. By streamlining these processes, AI can save instructors significant time, enabling them to focus more on improving assessment quality and giving effective feedback.
Furthermore, AI-driven adaptive assessments can adjust the difficulty level of tasks and assessments based on students’ responses. This approach offers a personalized evaluation experience and provides a more accurate picture of students’ knowledge, skills, and competencies.
However, despite its advantages, there are serious limitations to using AI for assessment and grading.
5.6 Revising methods of assessment and grading
Caveat: Follow the provisions of the EU AI Act
In the European Union, the AI Act plays a critical role in regulating the use of AI in teaching, particularly for testing and assessment purposes.
The AI Act explicitly identifies high-risk systems in the education sector (European Commission, 2024). These include AI applications related to admissions, performance assessment, educational standards evaluation, and monitoring during examinations. Performance assessment also encompasses systems designed to control the learning process.
AI applications explicitly intended for assessing examination papers are deemed particularly critical. Even the use of general-purpose AI for examination assessments is subject to stringent risk management requirements, including transparency, thorough documentation, and human oversight.
As a result, using AI for high-stakes decisions, such as course admissions or automated examination assessments, is discouraged for practical and ethical reasons. Universities must carefully evaluate whether deploying high-risk AI applications aligns with their responsibilities and regulatory obligations.
Revise your assessment methods and criteria
If AI is included as a means of learning and working on assignments and assessments, existing assessments are highly likely in need of improvement.
When revising your assessments, develop a clear system for criteria that ensures careful
-
distinction between different skill levels of AI usage according to your learning outcomes,
-
identification of different quality levels of practical work with AI,
-
identification of different compliance levels with standards and criteria of academic integrity and good scientific practice.
When students must use AI for examinations (written or oral), think about
-
how your examination questions and examination tasks must be designed to capture independent knowledge, skills, and competencies,
-
how students must document AI- and non-AI parts of assessments and how to explain this to students,
-
and how AI could assist you in correction and grading in a legally compliant way.
Strengthen competency-based assessment
Teachers realize that AI enables and requires assessments focused on skill mastery rather than memorization, tracking students’ competencies and skills in real-time. This shift encourages students to demonstrate understanding through the application and elaboration of personal approaches and results, rather than relying solely on traditional testing.
Hence, teachers need to develop further assessment methods that meet three main objectives:
- First, to prevent students from passing off AI-generated work as their own. This requires cross-referencing documentation and ensuring transparency.
-
Second, design assignments and examinations in such a way that the use of AI is documented in a way that it is possible to distinguish between AI-generated and independent performance parts.
-
Third, to develop examination methods that require students to practically demonstrate the knowledge and skills they have acquired, as well as to explain the solutions they have developed components.
Teachers are also increasing the oral or performative components of examinations in order to make individual learning progress and performance more visible. Here, it is very helpful to ask students to explain in more detail how they arrived at their results and to justify the choices they made.
Teachers should bear in mind, however, that increased oral examinations also lead to more time being spent on examinations.
Ultimately, it is clear that teachers need to refine three key elements of assessments when using AI in courses:
-
examination methods
-
assessment criteria
-
time required for implementing refined examinations
5.7 Demanding academic integrity and good scientific practice
Demand compliance with standards of independent scientific working
With generative AI tools capable of generating text, images, and code, academic institutions need to re-emphasize the relevance of original work. This shift necessitates the development of new academic integrity policies focused on requiring students to disclose AI use, to quote text generated by AI properly, and to explain their own and AI-generated components of their work.
Teachers need to decide on the appropriate documentation to implement in their courses to clearly distinguish between student work and AI-generated content, thus ensuring fair assessments.
Define rules for students’ transparent use of AI
Provide students with clear instructions on how to document and cite their work with AI. This is important for students to learn how to use AI in compliance with standards and rules of academic integrity. It is also important to be able to distinguish between AI-generated outputs and personal achievement in student work and assessments.
Many universities have developed specific templates for acknowledgment statements, requiring students to document their use of AI by explaining, as minimal requirements,
-
which AI tools they used (name and version),
-
the purpose for using one or more AI tools (e.g, generating ideas for study and assessments, paraphrasing and summarising sources, translating or optimizing text, developing presentations etc.)
-
Provide feedback on your ideas and work, and help you improve it
-
the prompts, follow-up prompts, and other inputs provided to the tool,
-
and how they adopted the AI output in their assignments, examinations, or theses.
Many universities require from students to provide declaration statements to acknowledge any permitted use of AI tools and technologies.
Teachers are strongly advised to communicate these requirements to students at the beginning of a course and to revisit their relevance during the course.
Raise awareness for reproducibility as a main property of scientific work
Reproducibility, understood as the ‘ability of independent investigators to draw the same conclusions from an experiment by following the documentation shared by the original investigators’ (Gundersen 2021) is a key criterion of scientific work in various sciences such as mathematical, physical, and engineering sciences. In other sciences such as the social sciences and the humanities, the natural science concept of reproducibility is less practical due to the properties of data, research methodologies, and research paradigms (Moody et al. 2022).
Since AI-generated outputs often lack explainability, it is important to raise students’ awareness of the relevance of making results and the way they have been developed comprehensible for others. This also supports the students’ ability to double-check the parts they have done independently, and which are AI-generated. Overall, reflecting reproducibility or comprehensibility of results raises awareness that students have to take responsibility for the entire work or results.
Classroom reproducibility refers to the ‘ability of an instructor to easily regenerate the results and conclusions of a student report from the submitted materials’ (Bean 2023). This implies that students submit materials appropriately organized, enabling the teacher to evaluate the final results as well as the process they were developed (ibd.).
One approach gaining more importance when using AI in the classroom could be to require students not only to submit the final work but also to require documentation of the entire work process, including the use of AI in relevant work phases and its impact on the process.
5.8 Evaluating how AI works in courses
Designing courses is a great opportunity to think about how teaching with AI should work out. The most important question is: How well is AI supporting students in achieving learning objectives? Several universities have already adopted relevant questions in their course evaluation and are able.
However, standard course evaluation systems may not capture all relevant topics, especially when using AI for the first time in a course and some trial and error can be assumed. In this situation, teachers may develop and apply relevant methods and criteria on their own.
Regarding the main evaluation topics, ask at least how helpful students find instructions and explanations on
-
the purpose and the method of AI use,
-
the required documentation;
and ask for information on
-
their tool-related experiences,
-
the perceived impact of AI on their learning activities and assessments,
-
and on the students’ ability to distinguish between self-generated and AI-generated results,
It is often useful to combine standardized and non-standardized methods and to ask relevant questions at the end of a teaching unit where AI was used, while reflection of specific topics may require a longer time of user experience and a greater variety of experiences.
5.9 Selecting appropriate AI tools
Apply distinct criteria for the selection of AI tools
Some AI tools may be more suitable for your course than others due to technical reasons.
In addition, teachers have to select appropriate AI tools for educational purposes by applying legal, didactic, social and ethical criteria, such as:
-
Compliance with data protection regulations
-
Alignment of the AI tool with learning objectives
-
Equal accessibility for all students: Every student must be able to use the permitted or prescribed AI application under the same conditions.
-
Documentability of AI outputs
-
Potential of the AI tool to foster creativity in teaching and learning
Define which tools can or must be used:
Often it is advantageous to specify which AI tools students must use, rather than allowing them to decide themselves. Teachers should decide:
-
Which AI applications are permitted or prohibited for a certain task or assignment
-
Whether specific AI applications are mandatory
-
How students must document their use of AI, including purpose, form, and extent.
6. Legal compliance and ethical responsibility
6.1 Committing students to compliance with legal regulations
Teachers are obliged to use AI in compliance with the following regulations and inform students in courses about their mandatory nature for all users of AI.
In all courses, teachers and students:
1. commit to the data-protection-compliant use of AI and AI products
2. are responsible, within the scope of teaching, learning, and research activities, for independently checking the legal restrictions of the data (input) used for data processing with AI before processing the data
3. are particularly obliged to
-
not use data as prompts which is subject to copyright
-
not use prompts or parts of prompts owned by third parties
-
not insert into AI applications sensitive and/or personal data or data that violate privacy
-
protection rights which also include company-related data such as emails by companies or individuals, in-company drafts, etc.
-
not use AI applications for discriminatory, offensive, or illegal purposes
4. use only data that does not infringe upon the rights of others when using AI applications for teaching, learning, and research activities
6.2 Putting responsible and ethical use to practice
Many universities have created institutional statements on the ethical and responsible use of AI. Since developing compliance skills takes time, and learning is an ongoing process, teachers are recommended to explain such regulations in every course, even at the risk of being repetitive.
Regardless of institutional policies, the following topics should be discussed with students where appropriate (cf. Shalevska 2024, European Commission 2022):
-
Transparency and oversight: As many generative AI are developed and owned by corporations, it may be important to think about how the tools are trained or what safeguards are in place to protect users from inaccurate information or harmful interactions.
-
Privacy, data governance, and safety: These aspects concern two levels of teaching action.
-
The first addresses the need to evaluate the policies of AI providers and the features of their tools: How is user data or copyrighted material used, stored, or shared? Who has access to user data? Which personal data (e.g. submitted when signing in as a user) is used for training data or for other purposes?
-
The second addresses AI users employing data: Which data am I allowed to use, e.g. as a part of a prompt? Which legal provisions and ethical considerations must be observed?
-
-
Diversity, non-discrimination, and fairness: AI systems may show algorithmic biases that perpetuate or exacerbate social inequalities. Do AI tools demonstrate unfair bias in their outputs? Are diverse groups characterized and represented in a non-discriminatory way in AI outputs? Are AI tools universally accessible? Are there discriminatory biases in algorithms?
-
Societal and political impact: What safeguards are in place to prevent AI from being used to spread inaccurate or discriminatory content? What is the impact on specific social groups if we follow the suggestions or decisions generated by the AI directly? How does AI affect the work, autonomy, and societal participation of different social groups? Is AI used to violate political rights by distorting information or influencing it in favor of a particular view?
-
Environmental impact: Training of AI tools with large data sets requires more and more energy consumption. What is the environmental impact of this energy use?
7. Recommended AI tools for teaching and learning
See the comprehensive AI-HED List of recommended AI tools.
References
Anthropic (2023). Prompt engineering overview. https://docs.anthropic.com/en/docs/build-with-claude/prompt-engineering/overview
Bean, B. (2023). Teaching Reproducibility to First Year College Students: Reflections from an introductory data science course. Journal on Empowering Teaching Excellence, Fall 2023. https://uen.pressbooks.pub/jete7i2/chapter/3/
Biggs. J., Tang, C. (2011) Teaching for Quality Learning at University. New York: Open University Press. https://cetl.ppu.edu/sites/default/files/publications/-John_Biggs_and_Catherine_Tang-_Teaching_for_Quali-BookFiorg-.pdf
Chiu, K. F. (2024). Future research recommendations for transforming higher education with generative AI. Computers and Education: Artificial Intelligence, Volume 6, June 2024. https://doi.org/10.1016/j.caeai.2023.100197
Ennis, R. (1991) Critical Thinking: A streamlined conception. Teaching Philosophy, 14:1, March 1991. https://education.illinois.edu/docs/default-source/faculty-documents/robert-ennis/ennisstreamlinedconception_002.pdf
European Commission (2024) AI Act. https://eur-lex.europa.eu/eli/reg/2024/1689/oj
European Commission (2023) Digital Competence Framework for Citizens (DigComp). https://joint-research-centre.ec.europa.eu/scientific-activities-z/education-and-training/digital-transformation-education/digital-competence-framework-citizens-digcomp/digcomp-framework_en
European Union (2022) Final report of the Commission expert group on Artificial Intelligence and data in education and training. Executive Summary. https://doi:10.2766/557340
European Union Agency for Fundamental Rights (2022) Bias in algorithms. Artificial Intelligence and discrimination. https://fra.europa.eu/sites/default/files/fra_uploads/fra-2022-bias-in-algorithms_en.pdf
European Union (2015) ECTS Users’ Guide 2015. https://doi:10.2766/87192
Facione, P. A. (1990). Critical Thinking: A Statement of Expert Consensus for Purposes of Educational Assessment and Instruction: Executive Summary, The Delphi Report. Millbrae, CA: California Academic Press. https://www.researchgate.net/publication/242279575_Critical_Thinking_A_Statement_of_Expert_Consensus_for_Purposes_of_Educational_Assessment_and_Instruction
Gundersen, O. E. (2021) The fundamental principles of reproducibility. https://doi.org/10.1098/rsta.2020.0210
Harvard University (2023) How Generative AI Is Reshaping Education. Practical Applications for UsingChatGPT and Other LLMs. https://he.hbsp.harvard.edu/how-generative-ai-is-reshaping-education.html
KI Campus (2024) Prompt-Labor 2.0. https://ki-campus.org/courses/prompt-labor
Lee, D., Arnold, M., Srivastava, M., Plastow, K., Strelan, P., Ploeckl, F., Lekkas, D., Palmer, E. (2024). The impact of generative AI on higher education learning and teaching: A study of educators’ perspectives. Computers and Education: Artificial Intelligence, Volume 6, June 2024, 100221. [https://doi.org/10.1016/j.caeai.2024.100221]{.underline}
Lee, S. (2023). AI Toolkit for Educators. EIT InnoEnergy Master School Teachers Conference 2023. https://paradoxlearning.com/wp-content/uploads/2023/09/AI-Toolkit-for-Educators_v3.pdf
Maastricht University (2024) AI Prompt Library. https://www.maastrichtuniversity.nl/education/edlab/ai-education-maastricht-university/ai-prompt-library
McDonald, N., Johri, A., Ali, A., Collier, A. H. (2025). Generative artificial intelligence in higher education: Evidence from an analysis of institutional policies and guidelines. Computers in Human Behavior: Artificial Humans, Volume 3, March 2025. [https://doi.org/10.1016/j.chbah.2025.100121]{.underline}
metalab at Harvard (2025) AI Guide. https://aipedagogy.org/guide/
Mollick, E., Mollick, L. (2023) Assigning AI: Seven approaches for students with prompts. file:///C:/Users/dietmar.paier/Downloads/ssrn-4475995.pdf
Moody, J. W., Keister, L. A., Ramos, M. C. (2022). Reproducibility in the Social Sciences. Annu. Rev. Sociol. 2022. 48:65–85. https://doi.org/10.1146/annurev-soc-090221-035954
OECD (2024) The potential impact of artificial intelligence on equity and inclusion in education. OECD Artificial Intelligence Papers, August 2024, No. 23. file:///C:/Users/dietmar.paier/Downloads/15df715b-en.pdf
OpenAI (2023). Prompt engineering. https://platform.openai.com/docs/guides/prompt-engineering .
Paulson, E. (2024) Critical thinking with AI: 3 approaches. https://tlconestoga.ca/critical-thinking-with-ai-3-approaches
UNESCO (2009) Defining an Inclusive Education Agenda: Reflections around the 48th session of the International Conference on Education. https://unesdoc.unesco.org/ark:/48223/pf0000186807
University of Applied Sciences BFI Vienna (2024) Recommendations of the Academic Council on using AI in university teaching. Vienna, restricted access.
University of Edinburgh (2024) Examples of how to use ELM with prompts. https://information-services.ed.ac.uk/computing/comms-and-collab/elm/getting-access-and-examples/examples-of-how-to-use-elm-with-prompts
University of Stanford (n.y.) Exploring the pedagogical uses of AI chatbots. https://teachingcommons.stanford.edu/teaching-guides/artificial-intelligence-teaching-guide/exploring-pedagogical-uses-ai-chatbots
Wharton University (2024) Prompt: Student Exercises. https://ai-analytics.wharton.upenn.edu/generative-ai-labs/student-prompt/